DICOM Segmentation: Complete Guide to Medical Image Analysis [2024]
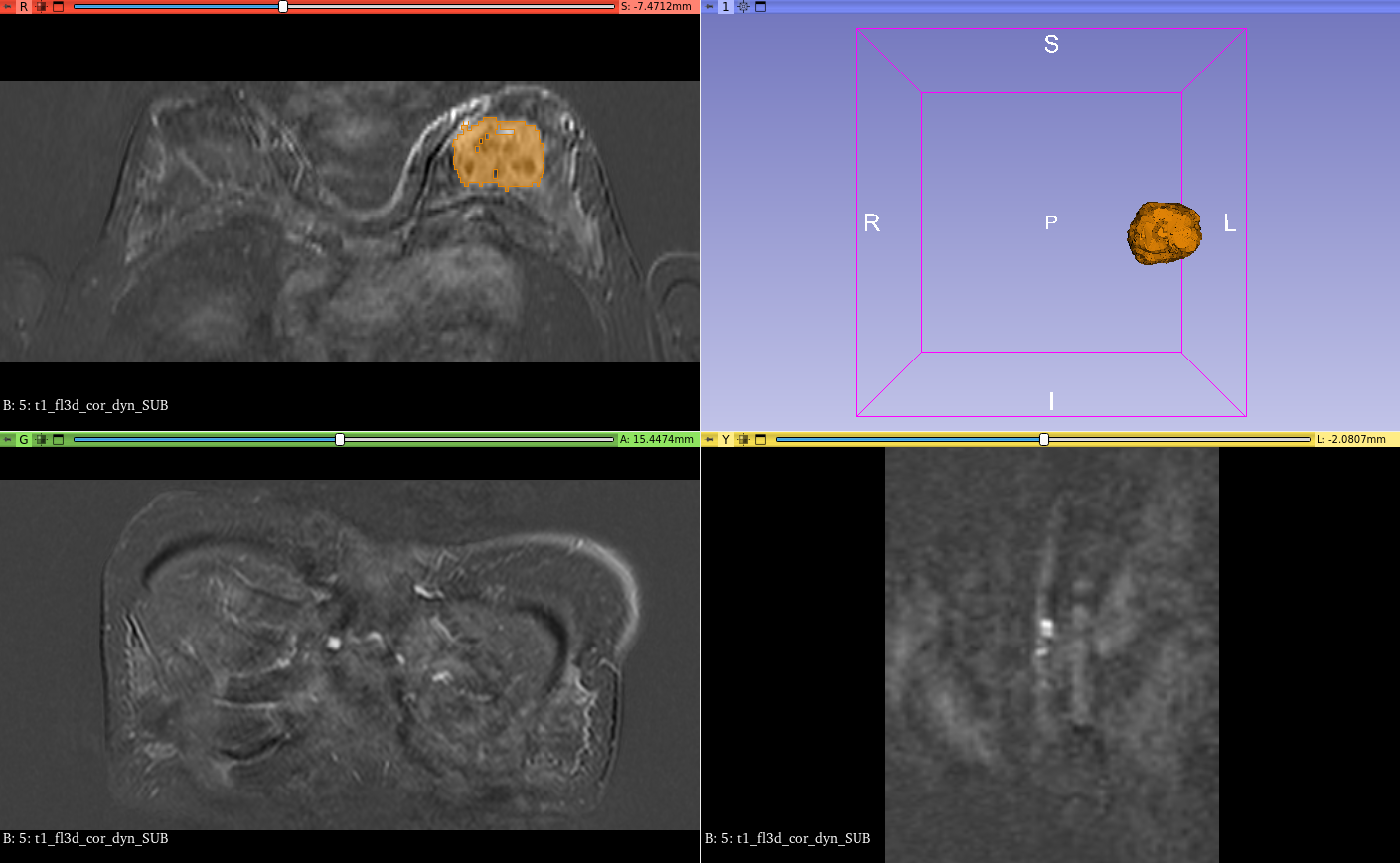
DICOM segmentation is a specialized medical imaging technique that classifies pixels in medical images using the DICOM standard. Medical professionals have multiple options for storing and managing segmentation data, primarily through DICOM SEG and DICOM RTStruct formats. Each format serves specific purposes in the medical imaging workflow, offering distinct advantages for different use cases.
What is DICOM Segmentation?
The medical imaging community has evolved to support multiple approaches to storing segmentation data. While both DICOM SEG and DICOM RTStruct serve the purpose of representing segmented regions in medical images, they were developed to address different clinical needs and workflows. Understanding their differences is crucial for implementing the right solution for specific medical imaging requirements.
DICOM SEG vs. RTStruct: Key Differences
DICOM segmentation can be implemented through two primary formats, each with its own strengths:
- DICOM SEG (Segmentation Storage SOP Class):
- Supports both binary and fractional segmentation
- Stores data as a multi-frame object
- Ideal for complex 3D segmentations
- Better suited for machine learning applications
- Maintains pixel-perfect accuracy
- DICOM RTStruct (Radiotherapy Structure Set):
- Originally designed for radiation therapy planning
- Uses contour-based representation
- More widely supported in legacy systems
- Lighter file size for simple segmentations
- Better for radiation therapy workflows
Also Read: What is DICOM? The Complete Guide to Medical Imaging Standards
Modern Segmentation Tools and Platforms
The landscape of medical image segmentation has evolved significantly with the introduction of advanced tools and platforms. These solutions combine the power of traditional DICOM formats with modern user interfaces and AI-assisted capabilities. One notable example is the Smart Paint tool by Collective Minds Research, which has revolutionized the interactive segmentation process for medical images.
Smart Paint and Advanced Segmentation
Smart Paint represents a significant advancement in medical imaging technology, offering an intuitive approach to segmentation that works with the RTStruct format for it's wide compatibility with legacy and open source software. The tool was notably utilized in the Horizon 2020 EuCanImage project, demonstrating its capability to handle large-scale segmentation tasks across thousands of medical examinations.
Also Read: DICOM Metadata Extraction: A Comprehensive Guide for Medical Imaging Professionals
Technical Implementation Considerations
When implementing DICOM segmentation in clinical workflows, several factors should be considered:
- For DICOM SEG:
- Higher storage requirements
- Better support for AI/ML workflows
- More detailed representation of segmented regions
- Superior handling of 3D volumes
- For DICOM RTStruct:
- Efficient storage for contour-based segmentations
- Widespread compatibility with radiation therapy systems
- Simpler data structure
- Easier integration with legacy systems
Also Read: DICOM Modalities: A Comprehensive Guide to Medical Imaging Technologies
Best Practices for Implementation
When working with DICOM segmentation:
-
Choose the appropriate format based on:
- Clinical use case
- Storage requirements
- System compatibility
- Workflow integration needs
-
Consider hybrid approaches:
- Use RTStruct for radiation therapy planning
- Implement SEG for advanced analysis and AI applications
- Maintain conversion capabilities between formats
-
Ensure proper validation:
- Verify segmentation accuracy
- Validate format compatibility
- Test system integration
"Radiologists in the hospital need to visualize and explore the images, identify where the
tumors and the lesions are, and label them. We face a problem of different vendors, different protocols, different scanners, different imaging contrast media, for the huge dataset of almost 25,000 Patients."Dr. Karim Lekadir is the Director of the Artificial Intelligence in Medicine Lab at the Universitat de Barcelona (BCN-AIM) and the Project Coordinator of EuCanImage
Summary
DICOM segmentation, whether implemented through SEG or RTStruct formats, provides essential functionality for medical image analysis. The choice between formats depends on specific clinical needs, system requirements, and workflow considerations. Modern tools like Smart Paint demonstrate how these traditional formats can be enhanced with contemporary user interfaces and AI assistance, improving both efficiency and accuracy in medical image analysis.
Introducing Collective Minds Research for Academia
FAQ
Which format should I choose: DICOM SEG or RTStruct?
The choice depends on your specific use case. Choose DICOM SEG for complex 3D segmentations and AI workflows, and RTStruct for radiation therapy planning or when working with legacy systems.
Can I convert between DICOM SEG and RTStruct formats?
Yes, conversion between formats is possible, though some information may be lost due to the different ways each format represents segmentation data.
How does Smart Paint work with DICOM segmentation?
Smart Paint provides an intuitive interface for creating segmentations that can be saved in either DICOM SEG or RTStruct format, depending on the clinical requirements.
What are the storage implications of each format?
RTStruct typically requires less storage space as it stores contours, while DICOM SEG stores full volumetric data, resulting in larger file sizes but more detailed representation.
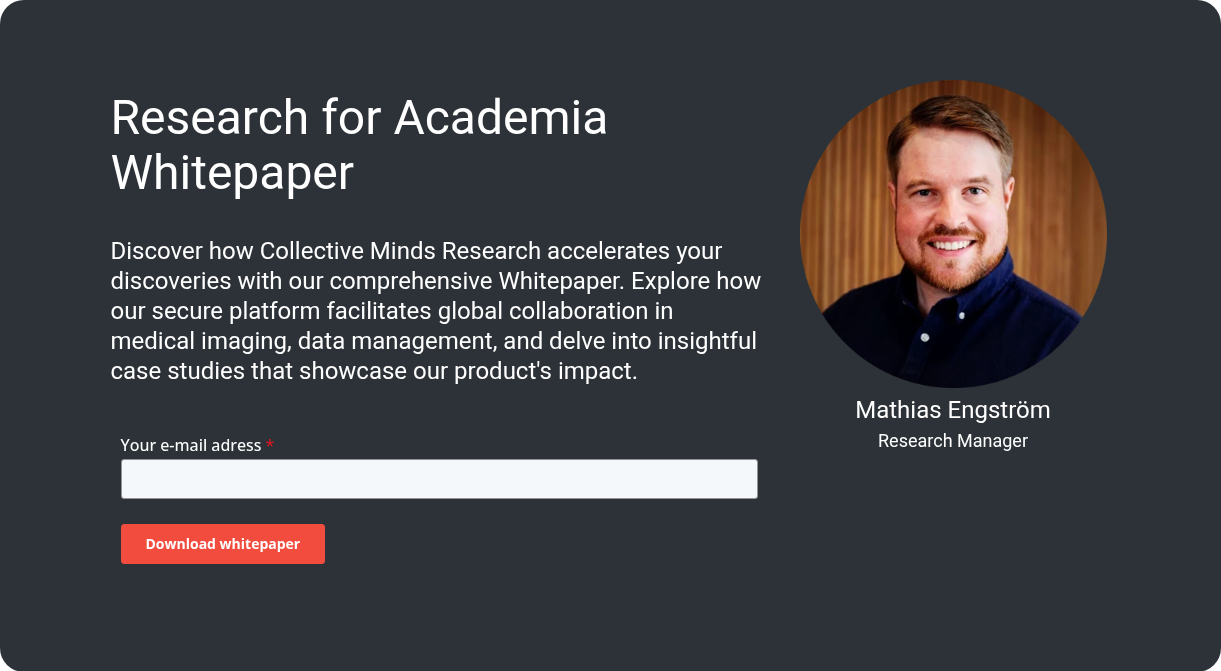
Reviewed by: Mathias Engström on November 14, 2024